We’re not trying to replace expertise—we’re trying to amplify and scale it. AI wants to create the expertise; we want to make yours omnipresent.
– Carl Wocke
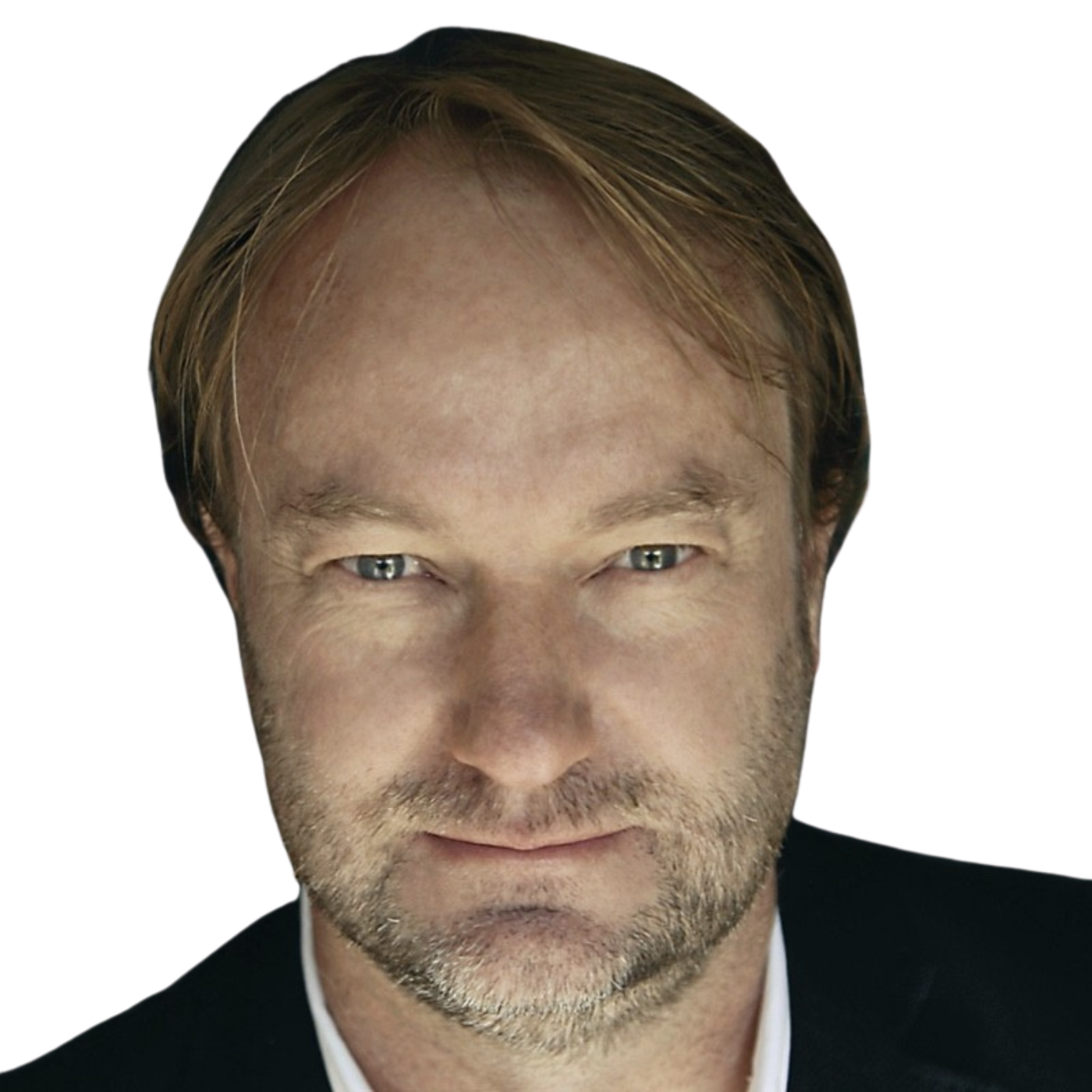
About Carl Wocke
Carl Wocke is the Managing Director of Merlynn Intelligence Technologies, which focuses on human to machine knowledge transmission using machine learning and AI. Carl consults with leading organizations globally in areas spanning risk management, banking, insurance, cyber crime and intelligent robotic process automation.
What you will learn
- Cloning human expertise through AI
- How digital twins scale decision-making
- Using simulations to extract tacit knowledge
- Redefining employee value with digital models
- Ethical dilemmas in ownership and bias
- Why collaboration beats data sharing
- Keeping humans relevant in an AI-first world
Episode Resources
Transcript
Ross Dawson: Carl, it’s wonderful to have you on the show.
Carl Wocke: Thanks, Ross.
Ross: So tell me about what Merlynn, your company, does. It’s very interesting, so I’d like to learn more.
Carl: Yeah. So I think the most important thing when understanding what Merlynn is about is that we’re different from traditional AI in that we’re sort of obsessed with the cloning of human expertise.
So where your traditional AI looks at data sources generating data, we are passionate about cloning our human experts.
Ross: So part of the process, I gather, is to take human expertise and to embed that in models. So can you tell me a bit about that process? How does that happen? What is that process of—what I think in the past has been called knowledge engineering?
Carl: Yeah. So we’ve built a series of technologies. The sort of primary technology is a technology called Tom. And Tom stands for Tacit Object Modeler.
And Tom is a piece of AI that has been designed to simulate a decision environment. You are placed as an expert into the simulation environment, and through an interaction or discussion with Tom, Tom works out what the heuristic is, or what that subconscious judgment rule is that you use as an expert.
And the way the technology works is you describe your decision environment to Tom. Tom then builds a simulator. It populates the simulator with data which is derived from the AI engine, and based on the way you respond, the data evolves.
So what’s happening in the background is the AI engine is predicting your decision, and based on your response, it will evolve the sampling landscape or start to close up on the model.
So it’s an interaction with a piece of AI.
Ross: So you’re putting somebody in a simulation and seeing how they behave, and using their behaviors in that simulation to extract, I suppose, implicit models of how it is they think and make decisions.
Carl: Absolutely so absolutely. And I think there’s sort of two main things to consider.
The one is Tom will model a discrete decision. And a discrete decision is, what would Ross do when presented with the following environment? And that discrete decision can be modeled within an hour, typically.
And the second thing is that there’s no data needed in the process. Validation is done through historical data, if you like. But yeah, it’s an exclusive sort of discussion between you and the AI, if that makes sense.
Ross: So when people essentially get themselves modeled through these frameworks, what is their response when they see how the model that’s being created from their thinking responds to decision situations?
Do they say, “These are the decisions I would have made?” I suppose there’s a feedback loop there in any case. But how do people feel about what’s been created?
Carl: So there is a feedback loop. Through the process, you’re able to validate and test your digital twin. We refer to the models that are created as your digital twin.
You can validate the model through the process. But what also happens—and this is sort of in the early days—is the expert might feel threatened. “You don’t need me anymore. You’ve got my decision.”
But nothing could be further from the truth, because that digital twin that you’ve modeled is sort of tied to you. It evolves. Your decisions as an expert evolve over time. In certain industries, that happens quicker.
But that digital twin actually amplifies your value to the organization. Because essentially what we’re doing with a digital twin is we’re making you omnipresent in an organization—and outside of the organization—in terms of your decisions.
So the first reaction is, “I’m scared, am I going to have a job?” But after that, as I said, it amplifies your value to the organization.
Ross: So one of the things to dig into there—here—but let’s dig into that for now, which is: what are the mechanics?
There are some ways we can say, “All right, my expertise is being captured,” and so then that model can do that work, not me. But there are other mechanisms where it amplifies value by, as you say, being able to be deployed in various ways.
So can we unpack that a little bit in terms of those dynamics of value to the person whose expertise has been embodied in a digital twin?
Carl: Yeah, Ross, that’s really sort of a sensitive discussion to have, in that when someone has been digitized, the role that they play in the organization is now able to potentially change.
So we have customers—banking customers—that have actually developed digital twins of compliance expertise. Those compliance experts can now go and work at the clients of the bank.
So the discussion or the relationship between the employer and the employee might well need to be revisited within the context of this technology. Because a compliance expert at a bank knows that they need to work the following hours, they have the following throughput. They can now operate anywhere around the world, theoretically.
So the value to the expert within a traditional corporate environment—or employee-employee environment—is going to be challenged. When you look at an expert outside of the corporate environment—so let’s say you’ve got someone who’s a consultant—they are able to digitize themselves and work pretty much anywhere around the world, in multiple organizations.
So I do—we don’t have the answer. Whose IP is it? Is another question. We’ve had legal advice on this. Typically, the corporate who employs the employee would be the owner. But if the employee leaves the organization, what happens to the IP? What happens to the digital twin?
So as Merlynn, we’ve sort of created this stage. We don’t have the answers, but we know it’s going to get interesting.
Ross: Yeah. So Gartner predicted that by 2027, 70% of organizations will be putting something in their employee contracts about AI representations, if I remember the statistics correctly.
And then I suppose what the nature of those agreements are is, as you say, still being worked out. And so these are fraught issues. But I think the first thing is to resurface them and be clear that they are issues, and so that they can be addressed in a way which is fair for the individuals as well as the organizations.
Carl: I think, Ross, just to add to that as well—the placement of the digital twin is now able to be sort of placed at an operational level, which also changes the profile of work that the employee typically has.
So that sort of feeds the statement around being present throughout the organization. So the challenges are going to be, well, I’m theoretically doing a lot more, and therefore I understand the value I’m contributing.
But yes, absolutely an interesting space to watch right now.
Ross: And I think there’s an interesting point here where machine learning is domain-bounded based on the dataset that it has been trained on.
And I think that any expertise from an individual—I mean, people, of course, build a whole body of expertise in a particular domain because they’ve been working, essentially—but what they have also done at the same time is enhanced their judgment, which I would suggest is almost always cross-domain judgment.
So a person’s judgment is still something they can apply across multiple domains. You can embody it within a specific domain and capture that in a system, but still, the human judgment is—and will remain, I think, indefinitely—a complement to what any AI system can do.
Carl: Absolutely. I think when you look at the philosophical nature of expertise, an expert—and this is sort of the version according to Carl here—is someone who cannot necessarily and readily explain their expertise.
If you could defend your expertise through data, then you wouldn’t be needed anymore, and you wouldn’t actually be an expert anymore. So an expert sort of jumps the gaps that we have within data.
What we found—and Merlynn has been running as an AI business for the last nine, ten years now, so we’ve been in the space for a while—is that the challenge with risk is that risk exists because I haven’t got enough data. And where I have a risk environment, there’s a drain on the expertise resource.
So experts are important where you have data insufficiency. So absolutely, to your point, I think the nature of expertise—when one looks at the value of expertise, specifically when faced with areas that have inherent risk—we cannot underestimate the value of someone making that judgment call.
Ross: So to ground this a little bit, I know you can’t talk too much about your clients, but they include financial services, healthcare, and intelligence agencies around the world. And I believe you have come from a significantly risk background.
So without necessarily being too explicit, what are some examples of the use cases, or where the domains in which organizations are finding this useful and relevant—and the match for the ability to extract or distill expertise?
Carl: So we focused on four main areas as a business, and these are areas that we qualify because they involve things that need to be done.
As a business, we believe it makes business sense to get involved in things that the world needs help with. So we focused on healthcare, banking, insurance, and law enforcement.
I’ll speak very high-level on all of these.
In healthcare, we’ve deployed our technology over the last four or five years, creating synthetic or digital doctors making critical decisions. In the medical environment, you can follow a textbook, and there’s a moment where you actually need a second opinion or you need a judgment call.
We never suggest replacing anything that AI is doing at the moment, or any of these phenomenal technologies. The LLMs out there—we think—are phenomenal technologies. We just think there’s a layer missing, which is: we’ve reached this point, and we’ve got to make that judgment call. We would value the input of a professor or an expert—domain expert.
So would there be benefit in that? In the medical space—treatment protocols, key decisions around being admitted—those are environments where you’ve got a protocol, but you don’t always get it right. And the value of a second opinion—our technology plays that second opinion role.
Where you’re about to do the following, but it might not be the correct approach.
In the medical world, there are two industries where we don’t think we’re going to make money, but we know we need to do it. And medical is one of them. Imagine a better world where we can have the right decision available at the right time, and we’ve got the technology to plan that decision.
So when you talk about telemedicine, you can now have access to a multitude of decisions in the field. What would a professor from a university in North America say?
Having said that, we work with the Emerys of the world—Emory Medical, Emory University—building these kinds of technologies.
So that’s medical.
On the insurance side, we’ve developed our technology to assist in the insurance industry in anything from claims adjudication, fraud, payments. You can imagine the complexity of decisions that are found within the processes in insurance.
In banking, we primarily focus on financial crime, risk, compliance, money laundering, terrorist financing-type interventions.
If I can explain the complexity of the banking environment: you’ve got all manner of AI technology that’s deployed to monitor transactions. A transaction is flagged, and that flagged transaction needs to be adjudicated by a human expert.
That’s quite telling of the state of AI, where you do all of the heavy lifting, but you have that moment where you need the expert. And that really is a bottleneck. Our technology clones your champion—or best-of-breed—expert within that space. You go from a stuck piece of automation to something that can actually occur in real time.
And then the last one is within the law enforcement space.
So we sponsor, here in South Africa, a very innovative collaboration environment, which comprises law enforcement agencies from around the world. We’ve got federal law enforcement agencies in North America. We’ve got the Interpols, Europols. We’ve got the Federal Police—Australian Federal Police—who participate.
So law enforcement from around the world, where we have created what they refer to as a safe zone, and where we have started to introduce our technology to see if we can help make this environment better.
The key being the ability to access expertise between the different organizations.
Ross: So in all of these cases that you are drawing—modeling—people who are working for these organizations, or are you building models which are then deployed more broadly?
Carl: Yeah, so in the line—well, in fact, across all of them—you know, there’s two answers to that.
The one is that organizations that deploy technology will obviously build a library of digital twin expertise and deploy that internally.
What we’re moving towards now is a platform that we’ve launched where organizations can collaborate as communities to fight, you know, joint risk. I’ll give you an example to sort of make that clearer.
So we won an innovation award with Swift. So Swift is a sort of a payments-type platform, monitoring-type platform. They’ve got many roles that they play. They’ve got 12,000 banks, and the challenge that they posed was: how do we get the banks to collaborate better?
And what we suggested was, if you attack one bank, what if you can draw on the expertise of the other banks? So if you’ve got a cyberattack or you’ve got some kind of financial crime unfolding, what if there’s a way for you to pool the expertise?
And I think that model allowed us to win that challenge, which answers the second part of the question, which is: do you bring expertise from outside of the organization?
We see a future where collaboration needs to take place, where we face common risk, common challenges. So the answer is both.
Ross: Yes, I can. I mean, there are some analogs of federated data, where you essentially take data which is not necessarily exposing it fully but be able to structure it so that’s available as a pool—for example, the MELLODY Consortium in healthcare.
But I think there are other ways. And so there’s Visa as well—it has some kind of a system for essentially sharing data on risk, which is aggregated and made available across the network.
And of course, you know, there are then the choices to be made inside organizations around what you share to be available, what you share in an anonymized or hidden fashion, or what you don’t share at all.
And essentially, there’s more and more value in ecosystems. And I think I would argue there’s more and more value, particularly in risk contexts, to the sharing to make this valuable for everyone.
Carl: Ross, if I can just add to that, I mean, you can share data, which has got so many compliance challenges.
You can share models that you created with the data, which I think is being exploited or explored at the moment.
The third is, I can share my experts. Because who do you turn to when things go off script? My experts. So they’re all valid.
But the future—certainly, if we want to survive—I mean, we have sight of the financial crime that’s being driven out there. It’s a war. And at times I wonder if we’re winning the war.
So we have to, if we want to survive, we have to find ways to collaborate in these critical environments. It’s critical.
And yet, we’re hamstrung by not being able to share data. I’m not challenging that—I think it’s important that that is protected.
But when you can’t share data, what am I sharing?
I go to community meetings in the form of conferences, you know, from time to time, and share thoughts and ideas. But that’s not operational. It’s not practical.
So we have to share our experts.
As Merlynn, we see expertise—and that second-opinion, monitoring, judgment-type resource—as so critical. It’s critical because it’s needed when things go off script.
We have to share this. So, yeah.
Ross: Yeah. So, moving on to Step—you also have this concept, I’m not sure, maybe we’ve decided to put it in practice—of an AI employment agency. So what is that? What does it look like? What are the considerations in that?
Carl: Yeah. So, the AI employment agency is a platform that we’ve actually established. So, I’m going to challenge you on the word “concept”—the platform’s running. It’s not open to the public, but it’s a marketplace—an Amazon marketplace—of digital twins.
So if I want to hire a compliance officer, and I’m a bank here in South Africa, I can actually go and hire expertise from a bank in America. I can hire expertise from a bank in Europe.
So, the concept or the product of the AI employment agency is a platform which facilitates creation and consumption. As an expert, we see a future where you can create a digital version of your expertise. And as a consumer—being the corporates, in fact, I suppose individuals would also be consumers—at the moment it’s corporates, but corporates can come and access that expertise.
And a very interesting thing happens. I’ll give you a practical example out of a banking challenge.
Very often, a bank has a thing called a “spike,” which is a new name added to a world database that looks for the undesirables. The bank has got to check their client base for potential matches, and that’s an instant sort of drain on expert resource.
What you could do with the employment agency is I could hire an expert, bring them into the bank for the afternoon to solve the challenge, and then just as readily let them go—or fire them out of that process.
So I think, just to close off on that, the fascination for me is: as we get older, hopefully we get wiser, and hopefully we stay up to date. But that skill—what happens to that skill?
What if there’s a way for us to mobilize that skill and to allow people to earn off that skill?
So the AI employment agency is about digitizing expertise and making it available within a marketplace. We’re going to open it up probably within the next 12 months. At the moment, it’s operational. It’s making a lot of people a lot of money, but we’ve got to be very careful once we open the gates.
Ross: But I think one of the underlying points here is that you are pointing to this humans-plus-AI world, where these digital twins are complements to humans, and where and how they’re being deployed.
Carl: Yeah. I think the—you know, I often see the areas where we differ from traditional AI approaches. And again, not negating or suggesting that it’s not the approach.
But when you look at a traditional AI approach, the approach is to replace the function. So replace the function with an AI component. The function would be a claims adjuster. And the guardrails around that—that’s a whole discussion around the agentic AI and the concerns around that. It brings hallucination discussions and the like.
Our version of reality is—we’re dealing with a limitation around access to expertise, not necessarily expertise. Whereas AI wants to create the expertise, we want to amplify and scale the expertise.
So they’re different approaches to the same challenge. And what we found is that both of them can live in the same space. So AI will do its part, and we will bring the “What does Ross think about the following?” moment, which is that key decision moment.
Ross: So I guess one of the issues of modeling—creating digital twins of humans—is that humans are… they may be experts, but they’re also fallible. There are some better than others, some more expert than others, but nobody is perfect.
And as a—part of that is, people are biased. They have biases in potentially a whole array of different directions.
So does this—all of the fallibility and the bias and the flaws of humanity—get embedded in the digital twin? Or if so, or if not, how do you deal with that?
Carl: Well, Ross, you might lose a whole lot of listeners now, but bias is a—well, let’s look at expertise. Expertise is a point of view that I have that I can’t validate through data.
So within a community, they’ll go, “Carl’s an expert,” but we can’t see it in the data, and therefore he might be biased.
So the concept of expertise—I see the world through positive bias, negative bias. A bias is a position that you hold that, as I said, is not necessarily accepted by the broader community, and expertise is like that.
An expert would see something that the community has missed.
So, you know, I live in South Africa. If you stop on the side of the road, it’s probably a dangerous exercise. But if there’s an animal, I’m going to stop on the side of the road. And that might be a sort of bad bias, good bias.
“Why did you do that?”—you put your family at risk and all of those things. So I can play out a position on anything as being positive and negative.
But I think we’ve got to be very careful that we don’t dehumanize processes by saying, “Well, you’re just biased,” and I’m going to take you out of the equation or out of the process.
In terms of people getting it right, people getting it wrong, good day, bad day—our technology is deployed in terms of an ensemble approach, where you would have a key decision.
I can build five digital twins to check on each other and monitor it that way. You can build a digital twin to monitor yourself. So we’ve built trading environments where the digital twin will monitor you as the trader, given that you’re digital twinned, to see whether you’re acting out of sorts—for whatever reason.
So bias—as I said, I hope I haven’t alienated any of your listeners—but bias is a… we’ve got to be very careful that we don’t use whatever mechanism we can to get rid of anything that allows people to offer that expertise into a process or transaction.
Ross: Yeah, no. Well, that makes sense.
And I suppose what it points to, though, is the fact that you do need diversity—as in, you can’t just have a single expert. You shouldn’t have a single human.
You bring diverse—as diverse as possible—perspectives of humans together. And that’s what boards are for, and that’s why you’re trying to build diversity into organizations, so you do have a range of perspectives.
And, you know, as you say, positive or useful biases can be… the way you’re using the term bias is perhaps a bit different than others, in saying it is just something which is different from the norm.
And—well—I mean, which goes to the point of: what is the norm, anyway?
But I think what this points to then is, if we can have a diverse range of experts—be they human or digital twins—then that’s when you design the structures where those, whatever those distinctiveness—not using the word “bias”—but say, those distinctive perspectives can be brought together into a more effective framing and decision.
Carl: Absolutely, Ross If I can sort of jump in and give you an interesting dilemma—the dilemma of fair business is something that… fairness is going to be decided by your customer.
So the concept of actually having a panel of experts adjudicating your business—because they say they think that this is fair.
Look at an insurance environment. Imagine your customers are adjudicating whether you should have, in fact, paid out the claim—even though you didn’t. That’s a form of bias. It’s an interpretation or an expectation of a customer to a corporate.
So I think, again, it just reinforces the value of bias—or expertise-slash-bias—because at the end of the day, I believe organizations are going to be measured against fairness of trade.
Now for AI—imagine the difficulty to find data to define fairness. Because your fair is different from my fair. I have different fairness compared to my neighbor.
How are we going to define that?
So again, that means there are so many individual versions of this, which is why I use the example of: organizations should actually model their customers and place them as an adjudicator into their processes or into their organizations.
Ross: Yeah. Well, I think part of the point here is, in fact, since AI embodies bias—human bias—because it’s trained on human data, it basically embodies human biases or perspectives, whatever.
So this is actually helping us to surface some of these issues around just saying, “Well, what is bias?” There is—it’s hard to say there is any objective… you know, there are obviously many subjective views on what bias is or how it could be mitigated.
These are issues which are on the table for organizations.
So just to round out—where do you see… I mean, the horizons are not very far out at the moment because it is moving fast—but what do you see as sort of the things on your mind for the next little while in the space you’re playing?
Carl: So I think if I look at the—two things.
One thing that concerns me about the current technology drive is that we are building very good ways to consume things, but we’re not building very good ways to make things.
And what I mean by that is—we’ve got to find ways for us as humans to stay relevant. If we don’t, we’re not going to earn. It’s as simple as that. And if we don’t, we’re not going to spend.
So it’s a very simplistic view, but I think it’s critical. It’s critical for us to keep humans relevant. And I think people—humans—are relevant to a process. So we’ve just got to find a mechanism for them to keep that relevance.
And if you’re relevant, you’re going to earn.
I don’t see a world where you’re going to be fed pizzas under the door, and you’re going to be able to order things because everything’s taken care of for you. That just doesn’t stack up for me.
So I think that’s a challenge.
I think the moment that we’ve arrived at now—which is an important moment—is the moment of human-in-the-loop.
How do we keep people in the loop? And human-in-the-loop is the guardrail for the agentic AI, for the LLMs, the Gen AIs of the world. That’s a very, very important position we need to reinforce.
And when one reinforces human-in-the-loop, you also bring relevance back to people. And then you also allow things like empathy, fairness of trade, ethics—to start to propagate through technology.
So I think the future for me—you know, I get out of bed, and sometimes I’m really excited about what the technology landscape holds. And then I’m worried.
So I think it’s going to work out when people realize: what are we racing towards here?
So again, concepts like human-in-the-loop—the guardrails—that are starting to become more practical.
So today, I’m excited, Ross. And let’s see what the future holds.
Ross: Yes. And I think it’s out of shape, because if we read this with the human-first attitudes, I think we’ll get there.
So where can people go to find out more about your work?
Carl: So you can go to merlynn-ai.com—so it’s M-E-R-L-Y-N-N dash A-I dot com.
You can also mail me at Carl@merlynn-ai.com if you want to have a discussion.
And, you know, good old Google—there’s a lot of information about us on the web. So, yeah.
Ross: Fantastic. Thank you for your time and your insights, Carl. It’s a fascinating journey you’re on.
Carl: Thanks, Ross. Thanks very much.
Podcast: Play in new window | Download