“The floor is rising really fast. So if you’re not ready to raise the ceiling, you’re going to have a problem.”
– Nisha Talagala
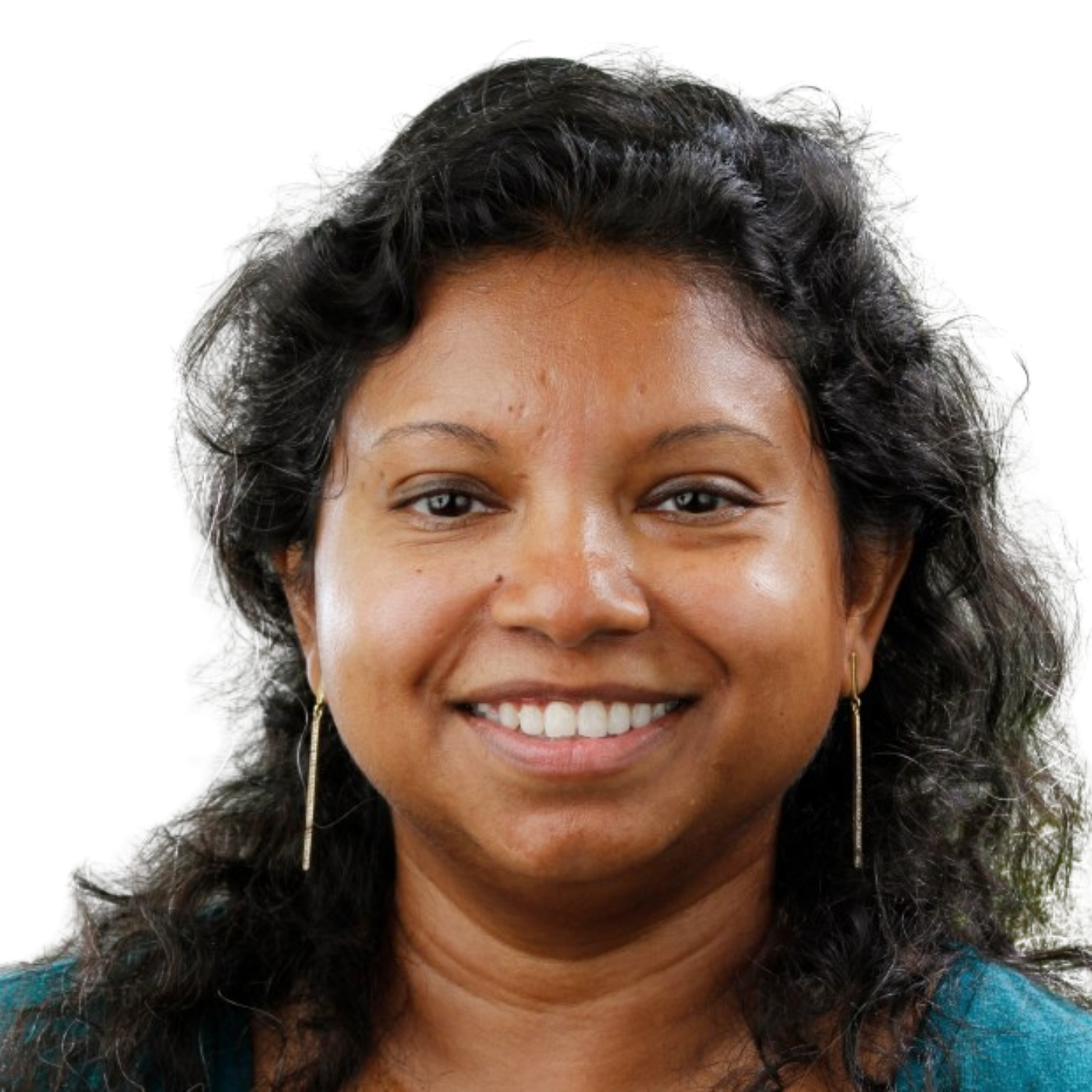
About Nisha Talagala
Nisha Talagala is the CEO and Co-Founder of AIClub, which drives AI literacy for people of all ages. Previously, she co-founded ParallelM where she shaped the field of MLOps, with other roles including Lead Architect at Fusio-io and CTO at Gear6. She is the co-author of Fundamentals of Artificial Intelligence – the first AI textbook for Middle School and High School students.
What you will learn
-
Understanding the four C’s of AI literacy
-
How AI moved from winter to wildfire
-
Teaching kids to build their own AI from scratch
-
Why professionals must raise their ceiling
-
The role of curiosity in using generative tools
-
Navigating context and motivation behind AI models
- Embracing creativity as a key to future readiness
Episode Resources
Transcript
Ross Dawson: Nisha, it’s a delight to have you on the show.
Nisha Talagala: Thank you. Happy to be here. Thanks for having me.
Ross: So you’ve been delving deep, deep, deep into AI for a very long time now, and I would love to hear, just to start, your reflections on where AI is today, and particularly in relation to humans.
Nisha: Okay, absolutely. So I think that AI has been around for a very long time. And there was a long time which was actually called AI winter, which is effectively that very few people working on AI—only the true believers, really.
And then a few things kind of happened. One of them was that the power of computers became so much greater, which was really needed for AI. And then the data also, with the internet and our ability to store and track all of this stuff, the data also became really plentiful.
So when the compute met the data, and then people started developing software and sharing it, that created kind of like a perfect storm, if you will. That enabled people to really see that AI could do things. Previously, AI experiments were very small, and now suddenly companies like Google could run really big AI experiments.
And often what happened is that they saw that it worked before they truly knew why it worked. So this entire field of AI kind of evolved, which is, “Hey, it works. We don’t actually know why. Let’s try it again and see if it works some more,” kind of thing.
So that has been going on now for about a decade. And so, AI has been all around you for quite a long time.
And then came ChatGPT. And not everyone knows, but ChatGPT is actually not the first version of GPT. GPT-1 and GPT-2 were pretty good. They were just very hard to use for someone who wasn’t very technical.
And so, for those who are technical—one thing is, you had to—actually, it was a little bit like Jeopardy. You had to ask your question in the form of an incomplete sentence, which is kind of fun in the Jeopardy sort of way. But normally, we don’t talk to people with incomplete sentences hoping that they’ll finish that sentence and give us something we want to know.
So ChatGPT just made it so much easier to use, and then suddenly, I think it just kind of burst on the mainstream. And that, again, fed on itself: more data, more compute, more excitement—going to the point that the last few years have really seen a level of advancement that is truly unprecedented, even in the past history of AI, which is almost already pretty unprecedented.
So where is it going? I mean, I think that the level—so it’s kind of like—so people talk a lot about AGI and generalized intelligence and surpassing humans and stuff like that. I think that’s a difficult question, and I’m not sure if we’ll ever know whether it’s been reached. Or I don’t know that we would agree on what the definition is there, to therefore agree whether it’s been reached or not reached.
There are other milestones, though. For example, standardized testing has already been taken over by AI. AI’s outperform on just about every level of standardized test, whether it’s a college test or a professional test, like the US medical licensing exam. It’s already outperforming most US doctors in those fields. And it’s scoring well on tests of knowledge as well.
And also making headway in areas that are traditionally considerably challenged—areas like mathematics and reasoning have become issues.
So I think you’re dealing with a place where, what I can tell you is that the AIs that I see right now in the public sphere rival the ability of PhD students I’ve worked with.
So it’s serious. And I think it’s a really interesting question of—I think the future that I see is that we have to really be prepared for tools that are as capable, if not in some areas more capable than we are. And then figure out: What is the problem that we are trying to solve in that space? And how do we work collaboratively with the tools?
I think picking a fight with the tools is unwise.
Ross: Yeah, yeah. And I guess my broader view is that the intent of being able to create an AI of humans as a reference point was always misguided. I mean to say, all right, we want to create intelligence. Well, the only intelligence we know is human, so let’s try to mimic that and to replicate what it does as much as possible.
But this goes to the point, as you mentioned, of augmentation, where on one level, we can say, all right, we can compare humans versus AI on particular tests or so on. But there are, of course, a multitude of ways in which AIs can augment humans in their capabilities—cognitive and intellectual and otherwise.
So where are you seeing the biggest potentials in augmenting intelligence or cognition or thinking or positive intent?
Nisha: Absolutely. So I think, honestly, the examples sort of—I feel like if you look for them, they’re kind of everywhere.
So, for example, just yesterday—or the day before yesterday—I wrote an article about vibe coding. Vibe coding is a term coined by Andrej Karpathy, which is essentially the way he codes now. And he’s a very famous person who, obviously, is a master coder. So he has alternatives—lots of ways that he could choose to write code.
And his basic point is that now he talks to the machine, and he basically tells it what he wants. Then it presents him with something. And then he says, “I like it. Change this, change that, keep going,” right?
And I definitely use that model in my own programming, and it works really well.
So really, it comes down to: you have something to offer. You know what to build. You know when you don’t like something, right? You have ideas. This is the machine that helps you express them, and so on and so forth.
So if you do that, that’s a very good way of doing augmented. So you’re creating something, and sometimes, when you see a lot of options presented to you, you’re able to create something better just because you can see it. Like, “Oh, it didn’t take me three weeks to create one. Suddenly I have fifteen, and now I know I have more cycles to think about which one I like and why.”
So that’s one example—just of creation collaboratively.
Examples in medicine just abound. The ability to explore molecules, explore fits, find new candidates for drugs—it’s just unbelievable.
I think in the next decade, we will see advancements in medicine that we cannot even imagine right now, just because of that ability to really formulate a problem, give a machine a task, have it come back, and then you iterate on it.
And so I think if we can just tap humans into that cycle and make that transition—so that we can kind of see a bigger problem—then I think there’s a lot of opportunity.
Ross: So, which—that leads us to the next thing. So the core of your work is around AI literacy and learning. And so it goes to the question of: AI is extraordinarily competent in many domains. It can augment us.
So what is—what are the foundational skills or knowledge that we require in this world? Do we need to understand the underlying architectures of AI? What do we need to understand—how to engage with generative AI tools?
What are the layers of AI literacy that really are going to be important in coming years?
Nisha: Very good question. So I can tell you that kind of early on in our work, we defined AI literacy as what we call the four C’s. We call them concepts, context, capability, and creativity.
Ross: Sorry, could you repeat this?
Nisha: Yes—concepts, context, capability, and creativity.
Ross: Awesome.
Nisha: So, concept is—you really should know something about the way these tools are created. Because as delightful as they are, they are not perfect. And a good user who’s going to use it for their own—who’s going to have a good experience with it—is going to be able to pick where and how to interact with it in ways that are positive and productive, and also be able to pick out issues, and so forth.
And so what I mean by concept is: the reliance of AI on data and being able to ask critical questions. “Okay, I’m dealing with an AI. Where did it get its data? Who built it? What was their motivation?”
Like these days, AIs are so complex that what I tell my students is: you don’t know what it’s trying to do. What is its goal? It’s sitting there talking to you. You didn’t pay for it—so what is it trying to accomplish?
And the easiest way to find out is: figure out who paid for it and figure out what it is they want. And that is what the AI is trying to accomplish. Sometimes it’s to engage you. Sometimes it’s to get information from you. Sometimes it’s to provide you with a service so that you will pay, in which case the quality of its service to you will matter, and such like that.
But it’s really important, when you’re dealing with a computer or any kind of service, that you understand the motivations for it. What is it being optimized for? What is it being measured on? And so forth.
So there’s kind of concepts like that—about how these tools are created. That does not mean everyone has to understand the nuances of how a neural network gets trained, or what it means to have a loss function, or all these things. That’s suitable for some people, but not necessarily for everyone.
But everyone should have some conceptual understanding.
Then context.
Ross: Or just gonna say, those interesting patterns on dark patterns. A paper in dark patterns on AI, which came out last week, I think, in one of the domains was second fancy, where, essentially, as you suggest, AI can say, “You’re wonderful” in all sorts of guises, which, amongst other things, makes you like it or more to use it more.
Nisha: Oh yes, they definitely have. They definitely want you to keep coming back, right?
You suddenly see that. And it’s funny, because I was having some sort of an interaction with—I’m not gonna name which company wrote the model—and it said something like, “Yeah, we have to deal with this.” And I’m like, there’s no we here. It’s just me. When did we become we?
You’re just trying just a little too hard to get on my good side here. So I just kind of noticed that. I’m like, not so good.
But so concepts, to me, effectively means that—underlying the fundamental ways that these programs are built, how they rely on data, what it means for an AI to have a brain—and then the depth depends entirely on the domain.
Context, for me, is really the fact that these things are all around us, and therefore you truly do want to know that they are behind some of the tooling that you use, and understand how your information is shared, and so forth.
Because there’s a lot of personal decisions to be made here, and there are no right answers. But you should feel like you have the knowledge and the agency to make your own choices about how to handle tools.
So that’s what I mean by context. It’s particularly important for young people to appreciate—context.
Ross: And I think for professionals as well, because their context is, you know, making decisions in complex situations. And if they don’t really appreciate the context—and the context of the AI—then that’s, that’s not a good thing.
Nisha: Absolutely.
And then capability—really, it varies very much on domain. But capability is really about: are you going to be able to function, right? Are you going to be able to do a project using these tools? Or do you need to build a tool? Do you need to merge the tools? Do you need to create your own tools?
So in our case, for young people, for example—because they don’t have a domain yet—we actually teach them how to build AI from scratch. So one of the very common things that we do is: almost in every class, starting from third grade, they build an AI in their first class completely from scratch. And they train it with their own data, and they see for themselves how its opinions change with the information they give it.
And that’s a very powerful exercise because—so what I typically ask students after that exercise is, I ask them two questions.
First question is: did it ever ask you if what you were teaching it was true? And the answer is always, no. You can teach it anything, and it will believe you. Because they keep teaching it information, and children being children, will find all sorts of hilarious things to teach a machine, right?
And then—but then—they realize, oh, truth is not actually a part of this.
And then the next question, which is really important, is: so what is your responsibility in this whole thing?
Your responsibility is to guide the machine to do the right thing, because you already figured out it will do anything you ask.
Ross: That’s really powerful. Can you tell me a little bit more about precisely how that works, and when you say, getting them to build their own AI?
Nisha: So we have built a tool. It’s called Navigator, and it’s effectively a web-based front end to industry standard tools like TensorFlow and scikit-learn. And it runs on the cloud.
Then we give each of our students accounts on it, and depending on how we do it, they can either—anonymized accounts, whatever we need to protect their privacy. At large-scale installations with schools, for example, it’s always anonymous.
Then what happens is they go in, and they’re taken through the steps of building an AI. We give them a few datasets that are kid-friendly. So one other thing to remember when you’re teaching young people is a lot of the data that’s out there is not friendly to young people, so we maintain a massive repository of kid-friendly datasets.
A very common case that they run is a crowdsourced dataset that we crowdsourced from children, which are sentences about happiness and sadness. So a child’s view—like chocolate might be happy, broccoli might be sad, things like that. But nothing sad—children can relate to.
So they start teaching about happy and sad. And one of the first things that they notice is—those of them that have written programs before—this is kind of hard to write a program for.
What word would you be looking for? There’s so many words. Like, I can’t use just the word happy. I might say, “I feel great.” I didn’t use the word happy, but I’m clearly happy. So they’re like, “Oh, so there’s something here—more than just looking for words. You have to find a pattern somehow.”
And if you give it enough examples, a pattern kind of emerges. So then they train the AI—it takes about five minutes. They actually load up the data, they train an AI, they deploy it in the cloud, and it presents itself as a little chatbot, if you will, that they can type in some sentences and ask it whether it thinks they’re happy or sad.
And when it’s wrong, they’re like, “Oh, it’s wrong now.” Then there’s a button they can press that says, “I don’t think you’re right.” And then it basically says, “Oh, interesting. I will learn some more.”
They can even teach it new emotions. So they teach it things like, “I’m hungry,” “I’m sleepy,” “I’m angry,” whatever it is. And it will basically pick up new categories and learn new stuff.
So after the first five minutes, when they interact with it—within about 15 minutes—every child has their own entire, unique AI that reflects whatever emotions they chose to teach and whatever perspective.
So if you want to teach the AI that your little brother is the source of all evil, then it will do that. And stuff like that.
And then after a while, they’re like, “Oh, I know how this was created. I can see its brain change.” And now you can ask it questions about what does this even mean when we have these programs.
Ross: That is so good.
Nisha: So that’s what I mean. And it has a wonderful reaction in that it takes away a lot of the—it makes it tangible. Takes away a lot of the fear that this is some strange thing. “I don’t know how it was made.”
“I made it. I converted it into what it is. Now I understand my agency and my responsibility in this situation.”
So that’s capability—and it’s also creativity in an element—because every single one of our projects, even at third grade, we encourage a creative use of their own choosing.
So when the children are very young, they might teach an AI to learn all about an animal that they care about, like a rabbit. In middle school, they might be looking more at weather and pricing and stuff like that.
In high school, they’re doing essentially state-of-the-art research. At this point, we have a massive number of high school students who are professionally published. They go into conferences and they speak next to PhDs and professors and others, and their work is every bit as good and was peer-reviewed and got in entirely on merit.
And that, I think, tells me what is possible, right? Because part of it is that when the tools get more powerful, then the human brain can do more things. And the sooner you put—
And the beautiful thing about teaching K–12 is they are almost fearless. They have a tremendous amount of imagination. They start getting a little scared around ninth grade—kicks in: “Oh, maybe I can’t do this. Maybe this isn’t cool. I’m going to be embarrassed in front of my friends.”
But before that, they’re almost entirely fearless. They have fierce imagination, and they don’t really think anything cannot be done. So you get a tool in front of them, and they do all sorts of nifty things.
So then I assume these kids, I’m hoping, will grow up to be adults who really can be looking at larger problems, because they know the tools can handle the simpler things.
Ross: That is, that is wonderful. So this is a good time just to pull back to the big picture of your initiatives and what you’re doing, and how all of these programs are being put into the world?
Nisha: Yeah, absolutely. So we do it in a number of different ways.
Of course, we offer a lot of programs on our own. We engage directly with families and students. We also provide curriculums and content for schools and organizations, including nonprofits. We provide teacher training for people who want to launch their own programs.
We have a professional training program, which is essentially—we work with both companies and individuals. In our companies, it’s basically like they run a series of programs of their choosing through us. We work both individually with the people in the company—sometimes in a more consultative manner—as well as providing training for various employees, whether they’re product managers, engineers, executives. We kind of do different things.
And then individuals—there are many individuals who are trying to chart a path from where they are to where—first of all, where should they be, and then, how can they get there? So we have those as well.
So we actually do it kind of in all forms, but we also have a massive content base that we provide to people who want to teach as well.
Ross: And so what’s your geographical scope, primarily?
Nisha: So we’re actually worldwide. The company—we started out in California. We went remote due to COVID, and we also then started up an office in Asia around that time. So now we’re entirely remote—everywhere in the world.
We have employees primarily in the US and India and in Sri Lanka, and we have a couple of scattered employees in Europe and elsewhere. And then most of our clients come from either the US or Asia. And then it’s a very small amount in Europe. So that’s kind of where our sweet spots are.
Ross: Well, I do hope your geographical scope continues to increase. These are wonderful initiatives.
Nisha: Thank you.Â
Ross: So just taking that a step further—I mean, this is obviously just this wonderful platform for understanding AI and its role in having development capabilities.
But now looking forward to the next five or ten years—what are the ways in which, for example, people who have not yet exposed themselves to that, what are the fundamental capability sets in relation to work?
So, I mean, part of this is, of course, people may be applying their capabilities directly in the AI space or technology. But now, across the broader domain of life, work—across everything—what are the fundamental capabilities we need?
I mean, building on this understanding of the layers of AI, as you’ve laid out?
Nisha: Yeah, so I think that, you know, a general sort of—so if we follow this sort of the four C’s model, right—a general, high-level understanding of how AI works is helpful for everyone.
And I mean, you know, and I mean things like, for example, the relationship between AI and data, right? How do AI models get created?
One of the things I’ve learned in my career is that—so there’s some sort of thing as an AI life cycle, like, you know, how does an AI get built? And even though there are literally thousands of different kinds of AI, the life cycle isn’t that different. There’s like this relationship between data, the models, the testing, the iteration.
It’s really helpful to know that, because that way you understand—when new versions come out—what happened. Yeah, what can you expect, and how does information and learning filter through?
You know, context is very critical—of just being aware. And these days, context is honestly not that complicated. Just assume everything that you’re—everything that you interact with—has an AI in it. Doesn’t matter how small it is, because it’s mostly, unfortunately, true.
The capability one is interesting. What I would suggest for the most broad-based audience is—really, it is a good idea to start learning how to use these foundation models. So I’m talking about the—you know—these models that are technically supposed to be good at everything.
And one of the things—the one thing I’ve kind of noticed, dealing with particularly professionals, is—sometimes they don’t realize the tool can do something because it never occurred to them to ask, right?
It’s one of those, like—if somebody showed you how to use the tool to, you know, improve your emails, right? You know the tool can do that.
But then you come along and you’re looking for, I don’t know, a recipe to make cookies. Never occurs to you that maybe the tool has an opinion on recipes for cookies. Or it might be something more interesting like, “Well, I just burned a cookie. Now, what can I do? What are my options? I’ve got burnt cookies. Should I throw out the burnt cookies? Should I, you know, make a pie out of them?”
Whatever it is, you know. But you can always drop the thing and say, “Hey, I burnt a cookie. Burned cookies.” And then it will probably come back and say, “Okay, what kind of cookies did you burn? How bad did you burn them?” You know, and this and that. “And here are 10 things you can do with them.”
So I think the simplest thing is: just ask. The worst thing it’ll do is, you know, it will come back with a bad answer. And you will know it’s a bad answer because it will be dumb.
So some of it is just kind of getting used to this idea that it really might actually take a shot at doing anything. And it may have kind of a B grade in almost anything—any task you give it.
So that’s a very mental shift that I think people need to get used to taking. And then after that, I think whatever they need to know will sort of naturally evolve itself.
Then from a professional standpoint, I think—I kind of call it surfing the wave. So sometimes people would come to me and say, “Hey, you know, I’m so behind. I don’t even know where to begin.”
And what I tell them is: the good news is, whatever it is that you forgot to look up is already obsolete. Don’t worry about it. It’s totally gone. You know, it doesn’t matter. You know, whatever’s there today is the only thing that matters. You know, whatever you missed in the last year—nobody remembers it anymore anyway.
So just go out there. Like, one simple thing that I do is—if you use, like, social media and such—you can tailor your social media feed to give you AI inputs, like news alerts, right, or stuff that’s relevant to you.
And it’s a good idea to have a feel for: what are the tools that are appropriate in your domain? What are other people thinking about the tools?
Then just, you know, pick and choose your poison.
If you’re a professional working for a company—definitely understand the privacy concerns, the legal implications. Do not bring a tool into your domain without checking what your company’s opinions are.
If the company has no opinions—be extra careful, because they don’t know, but they don’t know. So just—there’s a concern about that.
But, you know, just be normal. Like, just think of the tool like a stranger. If you’re going to bring them into the house, then, you know, use your common sense.
Ross: Well, which goes to the point of attitude. And part of it’s how—this—how do we inculcate that attitude of curiosity and exploration and trying things, as opposed to having to take a class, go in a classroom before you know what to do?
And you have to find your own path by—learn by doing. But that takes us to that fourth step of creativity, where—I mean, obviously—you need to be creative in how you try to use the tools and see what you learn from that.
But also, it goes back to this idea of augmenting creativity. And so, we need to be creative in how we use the tools, but also there are ways where we can hopefully create this feedback loop, where the AI can help us augment or expand our creativity without us outsourcing to it.
Nisha: Absolutely.
And I think part of this is also recognizing that—here’s the problem. If you’re—particularly if you’re a professional—this is less an issue for students because their world is not defined yet. But if you’re a professional, there is a ceiling of some kind in your mind, like “this is what I’m supposed to do,” right?
And the floor is wherever you’re standing right now. And your value is in the middle. The floor is rising really fast. So if you’re not ready to raise the ceiling, you’re going to have a problem.
So it’s kind of one of those things that is not just about the AI. You have to really have a mental shift—that I have to be looking for bigger things to do. Because if you’re not looking for bigger things to do, unfortunately, AI will catch up to whatever you’re doing. It’s only a matter of time.
So if you don’t look for bigger things—that’s why the areas that feel like medicine are flourishing—is because there are so many bigger problems out there.
And so, some of it is also looking at your job and saying, “Okay, is this an organization where I can grow? So if I learn how to use the AI, and I’m suddenly 10x more efficient at my job, and I have nothing left to do—will they give me more stuff to do?”
If they don’t, then I think you might have a problem.
And so forth. So it’s one of those—you have to find—there’s always a gap. Because, look, we’re a tiny little planet in the middle of a massive universe that we don’t know the first thing about. And as far as we know, we haven’t seen anyone else.
There are bigger problems. There are way, way bigger problems. It’s a question of whether we’ve mapped them.
Ross: Yeah, we always need perspective.
So looking forward—I mean, you’re already, of course, having a massive positive impact through what you are doing—but if you’re thinking about, let’s say, the next five years, since that’s already pretty much beyond what we can predict, what are the things that we need to be doing to shape a better future for humans in a world where AI exists, has extraordinary capabilities, and is progressing fast?
Nisha: I think really, this is why I focus so much on AI literacy.
I think AI literacy is critical for every single human on the planet, regardless of their age or their focus area in life. Because it’s the beginning. It’s going away from the fear and really being able to just understand just enough.
And also understanding that this is not a case where you are supposed to become—everyone in the world is going to become a PhD in mathematics. That’s not what I mean at all.
I mean being able to realize that the tool is here to stay. It’s going to get better really fast. And you need to find a way to adapt your life into it, or adapt it into you, or whichever way you want to do it.
And so if you don’t do that, then it really is not a good situation.
So I think that’s where I put a lot of my focus—on creating AI literacy programs across as many different dimensions as I can, and providing—
Ross: With an emphasis on school?
Nisha: So we have a lot of emphasis on schools and professionals. And recently, we are now expanding also to essentially college students who are right in the middle tier.
Because college students have a very interesting situation—that the job market is changing very, very rapidly because of AI. So they will be probably the first ones who see the bleeding edge. Because in some ways, professionals already have jobs—yes—whereas students, prior to graduating from college, have time to digest.
It’s this year’s and next year’s college graduates who will really feel the onslaught of the change, because they will be going out in the job market for the first time with a set of skills that were planned for them before this happened.
So we do focus very much on helping that group figure out how to become useful to the corporate world.
Ross: So how can people find out more about your work and these programs and initiatives?
Nisha: Yeah, so we have two websites.
Our website for K–12 education is aiclub.world. Our website for professionals and college students—and very much all adults—is aiclubpro.world. So you can look there and you can see the different kinds of things we offer.
Ross: Sorry, could you repeat the second URL?
Nisha: It’s aiclubpro.world.
Ross: aiclubpro.world. Got it?
That’s fantastic. So thank you so much for your time today, but also your—the wonderful initiative. This is so important, and you’re doing a marvelous job at it. So thank you.Â
Nisha: Really appreciate it. Thank you for having me.
Podcast: Play in new window | Download